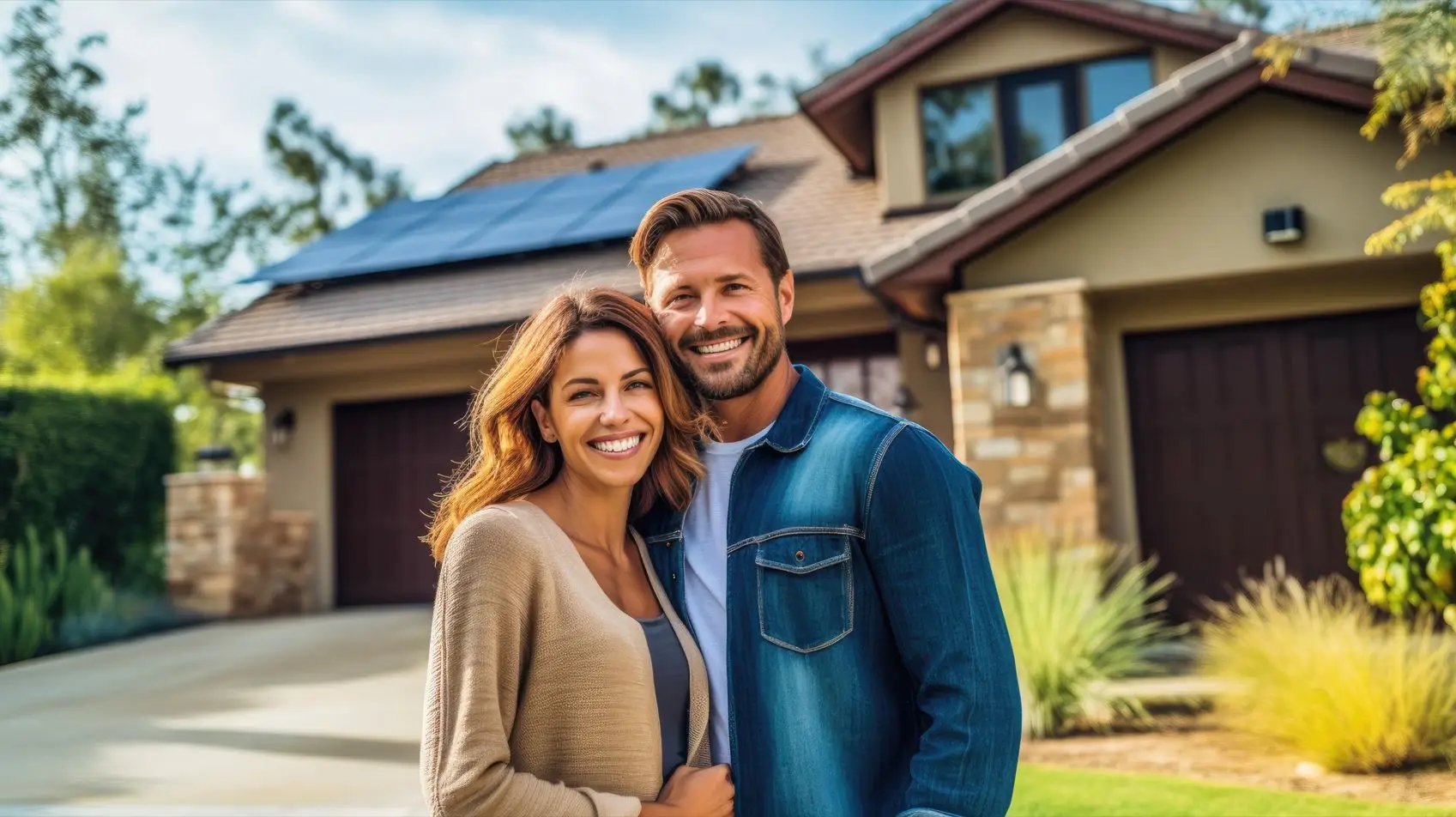
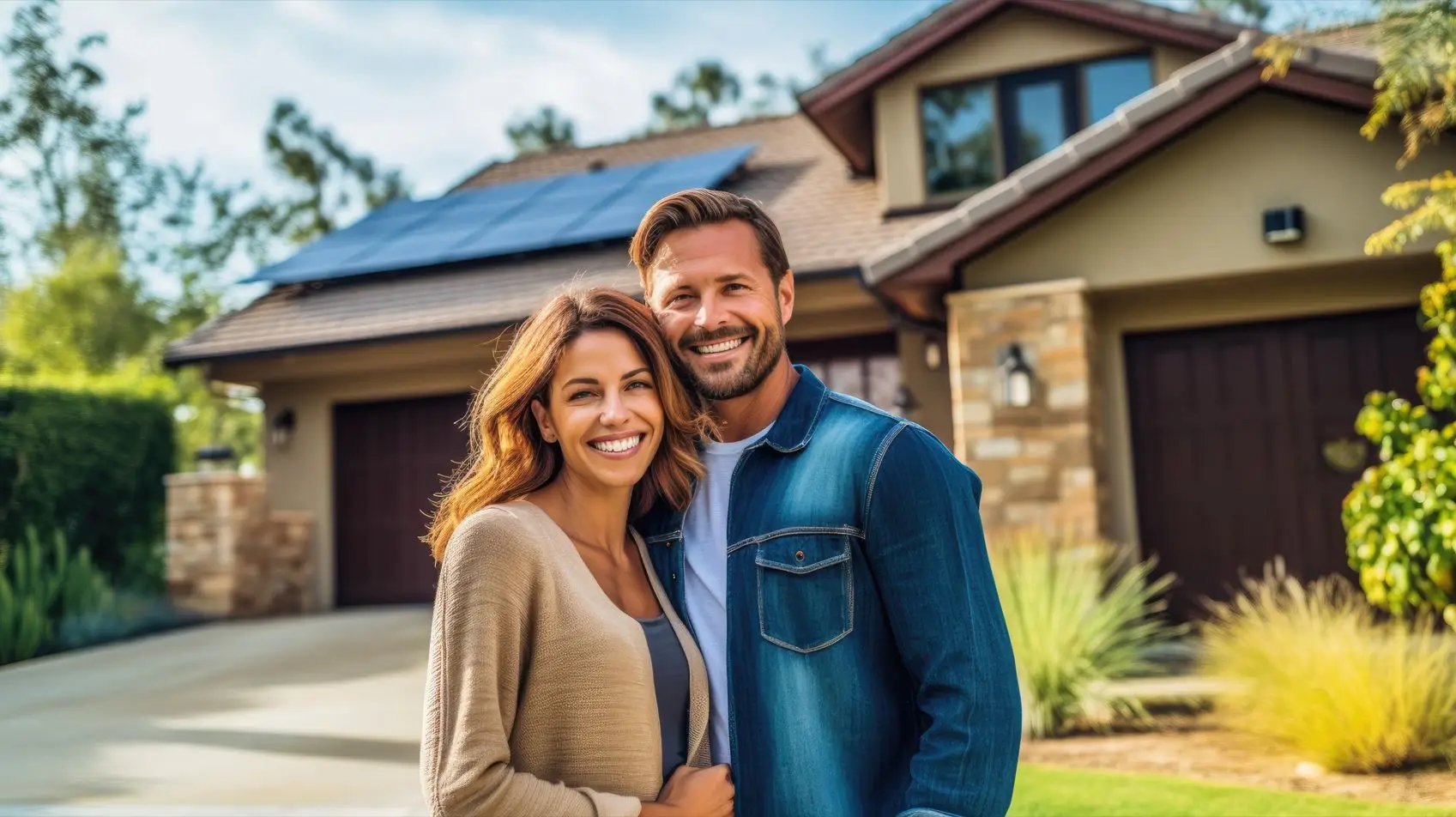
A group of researchers employed various machine-learning techniques and found that solar panel installations can significantly increase the home value of properties in the UK. The analysis incorporated property details from Zoopla, which is a significant provider of property listings in the UK, as well as price data from the governmental Land Registry, looking at the timeframe from 2012 to 2018.
Researchers in the UK employed a computational technique known as machine learning to investigate whether residences with solar panels command higher sale prices. They found that homes equipped with solar panels tend to sell for higher prices.
According to the researchers, there is a consensus that energy-efficient homes with a ‘green’ label are viewed favorably in the real estate market. There is a lack of extensive research on the effects of solar panels on homes, with the majority of existing information sourced from the United States and Australia. We are examining the impact of residential solar panels on property values in the UK, and there is a lack of prior research on this topic. The researchers utilized specialized software to examine approximately 5 million data entries related to homes sourced from Zoopla, a prominent property listing site in the UK, alongside price information from the government’s Land Registry spanning the years 2012 to 2018. To identify houses with solar energy, the algorithms were programmed with terms like “PV panel” and “solar” for sorting purposes. Following that, they managed to associate 80% of the listings with actual PPD.
According to the group, “In selecting the samples, we avoid including residences that have more than three bathrooms or exceed five bedrooms.” Since the apartments in the buildings share a roof, we will omit them from our research since the owners could face difficulties installing solar panels. Ultimately, we eliminate the properties with the top and bottom 1% of selling prices to filter out outlier data.
The researchers implemented five varied algorithms for their learning framework: the S-learner, T-learner, X-learner, R-learner, and DR-learner.In machine learning, a meta-learner refers to a technique that employs various base learners to improve the quality of its predictions. According to the researchers, both the S-learner and T-learner utilize only fundamental models, which contributes to their simplicity. The other three utilize additional details, resulting in a more complex interpretation.
The S-learner approach determined an average effect of 0.055. This indicates that homes equipped with solar panels have a selling price that is 5.5% higher. Implementing more sophisticated learning techniques results in an increase of over 6% in the value of solar panels. It increases from 6. 1% with the X-learner to 7. 1% with the DR-learner.
Upon further examination, the researchers discovered variations across various years, locations, and price ranges. They observed that the advantages provided by solar panels have diminished over time. The most favorable returns are found in Wales, Yorkshire, and the Humber, with the Northwest and Southwest areas following closely behind. According to their findings, solar panels have a more significant effect on the prices of both the least expensive and most expensive homes, with the highest price surges occurring in the priciest properties. The reduced prices available demonstrate that those with tight budgets can save on their energy bills and take advantage of lower costs. The elevated prices in the luxury sector may indicate a growing interest among consumers in investing in environmentally sustainable properties. This further highlights their passion for showcasing their efforts to protect the environment.
In order to evaluate the strength of the results, the new model was juxtaposed with traditional techniques, specifically the hedonic pricing model, and coarsened exact matching (CEM). The initial approach typically evaluates the extent to which each factor affects property prices, while the latter deals with real-world data. The scientists discovered that the hedonic pricing model indicated a 5. 6% increase in value, while the CEM showed a 3. 5% increase. They noted that the outcomes may vary depending on the techniques employed. They came up with the finding that the Metalearner algorithms are more effective than hedonic models in scenarios with intricate treatments, numerous variables, and subtle effects of property features on house prices, particularly in the presence of ambiguous influences.
They published their findings in a paper titled “Returns to Solar Panels in the Housing Market: A Meta Learner Approach,” which was featured in the journal Energy Economics.The team was composed of scholars from the University of Birmingham and Swansea University.